Are Call Center QA Programs Broken?
It is starting to be a well-accepted belief that call center Quality Assurance (QA) is broken and has little or no impact on improving Customer Satisfaction (Csat) and First Call Resolution (FCR). Furthermore, SQM Group's research shows that only 19% of managers strongly agree that their call center's quality assurance program helps improve Csat.
Interestingly, SQM has many clients who have stopped conducting QA evaluations for measuring call center customer service support, and it has had no negative impact on Csat. These same call centers have continued to use auto-compliance to evaluate 100% of the calls to ensure that their agents adhere to government laws and company policies with much success.
Call center QA practices currently being used by companies have many problems. From a company perspective, in many cases, it only evaluates 1-2% of customer calls and is a manual process driven by employees. In addition, QA evaluators can be inconsistent with their QA evaluation scoring, inefficient for evaluating calls, and expensive.
Most call center's QA program goals are to improve Csat, but that seldom occurs. SQM Group's research shows that 83% of agents do not believe their QA program helps them improve Csat or FCR. Moreover, only 15% of agents are very satisfied with QA monitoring, and it has been one of the drivers for agent turnover. In addition, 81% of agents' QA scores do not correlate with Csat or FCR scores. Put simply, traditional QA has no or limited impact on positively impacting Csat scores and FCR rates.
The impact of not achieving FCR on Csat, customer recommendation, and retention when a customer interacts with a call center is poorly understood. Did you know SQM Group reports that for every 1% improvement you make in FCR, you get a 1% improvement in customer satisfaction? Furthermore, FCR and Csat metrics have a +0.70 positive correlation coefficient. A metric correlation coefficient over +0.50 is considered strong.
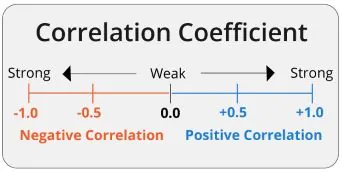
FCR Impact On Net Promoter Score
Also, customer recommendations (NPS) are 85 points or 5.7x higher when a CX was an FCR call than for an unresolved call. The graph below shows that NPS is 70 points when FCR is achieved and potential company promoters. Conversely, when customer calls are unresolved, the NPS is -15 points; when this occurrence takes place, it indicates these customers are potential detractors.
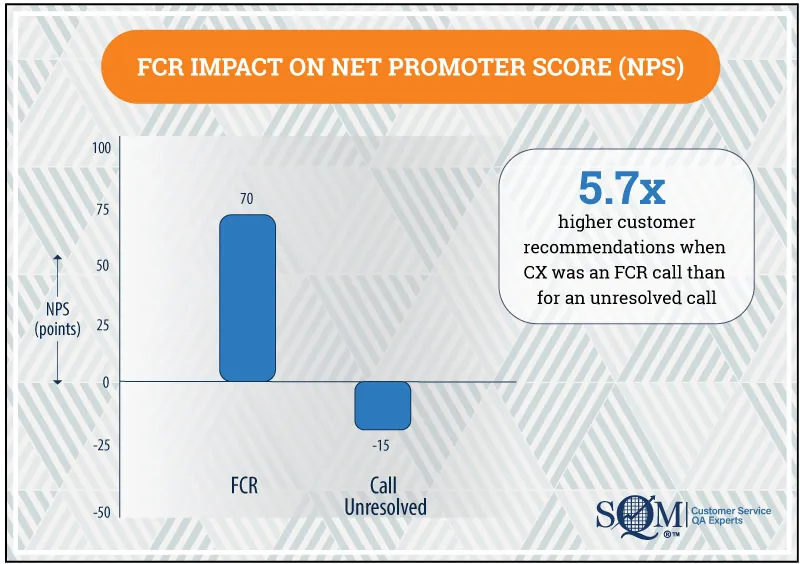
FCR Impact On Net Retention Index
Customer retention (NRI) is 70 points or 5.4x higher when a CX was an FCR call than for an unresolved call. The graph below shows that NRI is 85 points when FCR is achieved and indicates customer retention security. Conversely, when customer calls are unresolved, the NRI is 16 points; when this occurs, it indicates these customers have the potential for being at risk to defect to your competition.
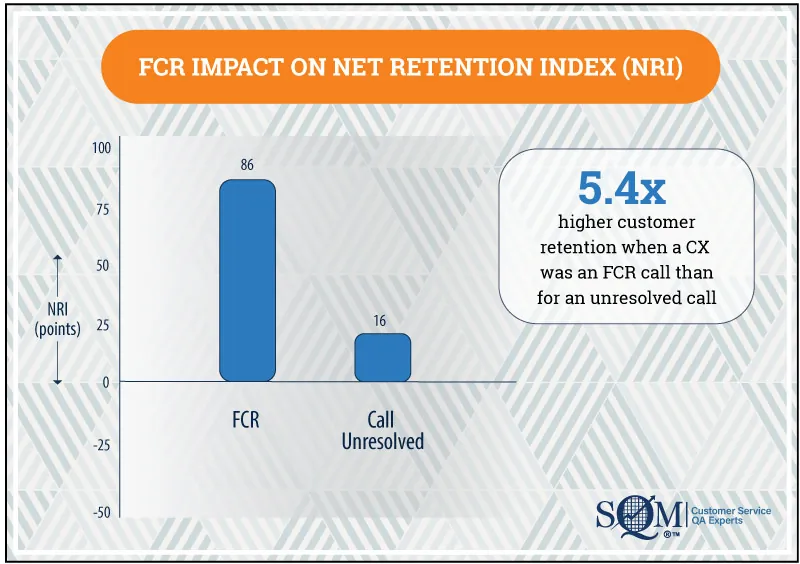
Why Is Call Center QA Broken?
Call center QA and compliance are related, but there are distinct differences. For example, the main goal of QA is to enhance the overall CX, improve agent performance, and maintain or enhance the brand's reputation. Conversely, the primary objective of compliance is to ensure that the call center operates within the boundaries of laws, company policies, and regulations to avoid legal issues, fines, and reputational damage.
The problem with call center quality assurance is QA evaluators are inconsistent, inefficient, and expensive; most call center QA programs do not help agents improve their QA performance for metrics such as Csat, FCR, and call resolution. However, it is fair to say that script compliance (e.g., adherence to government laws and company policies) insights have been successful in helping companies avoid legal issues, fines, and reputational damage.
Furthermore, most QA programs focus on the wrong metrics because they assume the QA metrics they are using help improve CX and, therefore, should positively impact Csat and FCR. For example, it is common for QA evaluators or Artificial Intelligence (AI) evaluations to measure if the agent used the customer's name multiple times during the call to make the customer feel the interaction was personalized and thus assumed it improved Csat. However, research shows that this call-handling technique has very little or no impact on Csat.
In addition, call center managers usually assume their QA scorecard measures the right metrics to drive improvement in FCR and Csat. However, they seldom or never verify if the QA metrics help improve FCR and Csat outcome metrics.
Enlightened call center managers resist prioritizing QA metrics that have not been validated to improve CX outcome metrics such as Csat. Instead, they focus on resolving a customer's inquiry or problem metric, which the SQM Group has validated to be the most important metric for positively impacting Csat.
Moments of Truth Importance Ranking
Below is SQM Group's research showing call center Moments of Truth (MoT) customer importance ranking based on a statistical correlation to Csat. The research shows that any MoT can impact the CX; however, any MoT over 0.50 correlation coefficient is statistically important to the customer's experience when calling the call center. Therefore, MoTs with over 0.50 correlation coefficient significantly impact Csat.
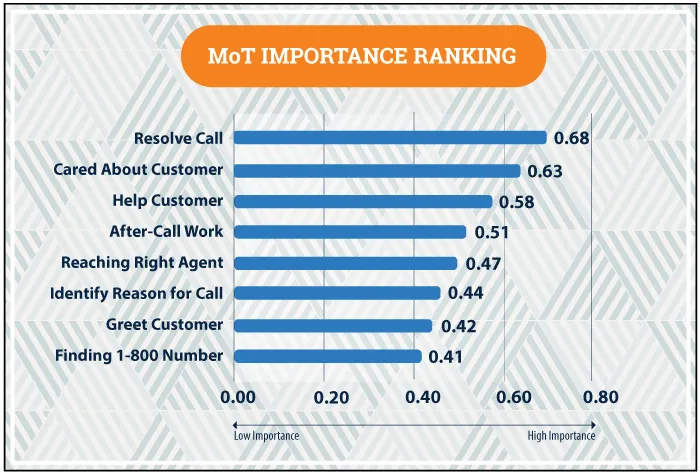
For example, the below graph shows the MoT that matters the most, from a customer's perspective, is call resolution (preferably on the first call), caring about the customer, helping the customer, and after-call work. These four MoT have a statistical correlation coefficient of .50 or higher, so they are considered the MoT that matter the most to driving high Csat.
QA assessment should include MoT metrics that customers view as important, such as call resolution, customer caring, and helping customers. MoTs QA metrics can be measured using post-call surveys and AI sentiment analysis methods to determine agent, team, and call center performance. All QA metrics should be correlated to Csat to validate that they have a positive impact on Csat.
Fix QA First, Then Use AI to Automate and Post-call Surveys to Validate
SQM Group is of the opinion that call centers need to fix QA first, then use AI to automate and post-call surveys to validate QA metrics impact for improving Csat. A major problem with QA not being effective for driving Csat is how QA is designed.
The QA design issues have been that most agents are evaluated on 4 to 10 calls per agent per month, which the majority of agents and supervisors view as a small sample size. Furthermore, in most cases, QA evaluators or supervisors can be inconsistent with their QA evaluation scoring, inefficient for evaluating calls, and expensive. Also, it is common for QA programs to use the wrong metrics that have little or no impact on Csat. Lastly, in many cases, supervisors are not effective in coaching agents to improve Csat.
AI is being used to automate QA and compliance processes so call centers can efficiently evaluate all calls, score calls more consistently, and reduce operating expenses. However, the bigger opportunity is to significantly fix the effectiveness of QA for improving Csat and FCR. Traditional QA metrics are determined based on biases, assumptions, and gut instincts. Furthermore, QA metrics are seldom validated to verify that they impact Csat positively.
To have the right QA metrics that drive Csat, you need to validate that the metrics being used on the agent QA scorecard are effective for creating high Csat. The use of AI sentiment analysis and post-call surveys are useful techniques for determining if a call center is using the right QA metrics for positively driving Csat.
AI sentiment analysis can determine the emotional tone of customer interactions, providing insights into whether customers had a positive, neutral, or negative experience. However, the best practice for validating if QA metrics are positively impacting Csat is the use of post-call surveying. It is important to emphasize that customers’ opinions matter the most, and the best approach to gathering their feedback is conducting post-call surveys.
SQM Group's research shows that call center professionals view the combination of post-call surveys and QA metrics as the best method for assessing and improving your call center's customer satisfaction performance. Furthermore, SQM reports that using the combination of surveys and QA has a proven track record for positively impacting Csat scores and is a comprehensive approach for evaluating calls.
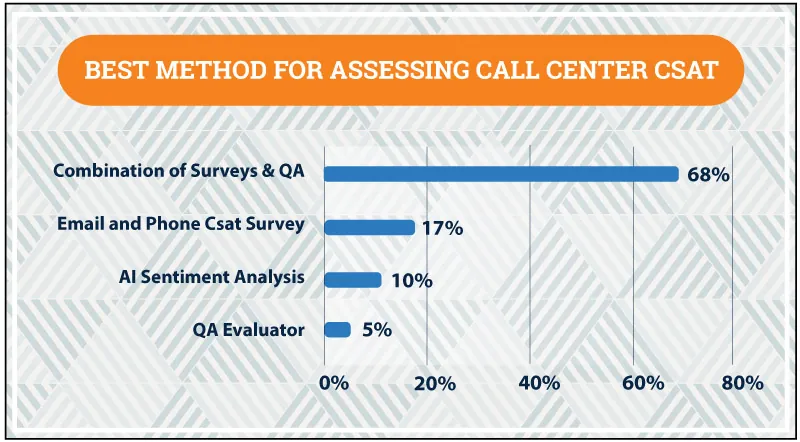
Ways That AI Can Be Applied to Automate 100% of the Evaluation of Calls to Make QA More Effective and Efficient
Auto-QA and compliance processes eliminate or significantly reduce manual QA evaluations by automating them. By scoring 100% of calls with AI, you can remove human errors and agent biases from the call center QA program. Auto-QA and compliance is a more effective way to manage QA and compliance because it dramatically increases the scale of QA evaluations by going from 1 to 2% up to 100%. Therefore, you can identify compliance issues in real time to take the necessary action.
Automating QA is becoming a common practice and has been effective in assessing and providing insights for managing compliance adherence. However, automating QA using AI sentiment analysis has been less successful in assessing CX and improving Csat, mainly due to not using the right QA metrics and not consistently aligning with the customer's viewpoint of how they assess their own CX.
Again, AI is being used to automate call center QA and compliance processes to a significant extent. This involves using various AI technologies and techniques to evaluate and assess the compliance adherence and quality of customer service agent's delivery. Here are some ways in which AI can be applied to automate call center QA and compliance:
- Speech Analytics: AI-powered speech recognition and natural language processing (NLP) algorithms can transcribe and analyze customer-agent interactions. AI can identify keywords, phrases, and sentiments in conversations, helping spot QA and compliance adherence opportunities for improvement.
- Sentiment Analysis: AI can determine the emotional tone of a conversation between agents and customers. Automatically categorize responses as positive, neutral, or negative to gauge customer satisfaction. Customer sentiment can help determine CX and assist in flagging calls for service recovery opportunities.
- Compliance Monitoring: AI can be used to ensure that agents adhere to government regulatory laws and company-specific compliance guidelines. It can flag instances where agents fail to adhere to scripts and policies or provide required disclosures to help avoid legal issues, fines, and reputational damage.
- Performance Metrics: AI algorithms or analytical tools can track and analyze various performance metrics, such as call resolution, call duration, hold times, helpdesk support, call transfers, and agent response times, to assess efficiency and effectiveness.
- Automated Scoring: AI or analytical tools can provide automated scoring for calls based on predefined criteria and benchmarks. This can include scoring for QA and compliance metrics to determine a total QA score.
- Trend Analysis: Over time, AI can help call centers identify trends and patterns in customer interactions, which can be valuable for making strategic and operational decisions and improving customer service at the agent and call center levels.
- Quality Feedback: AI can generate automated feedback reports for agents, highlighting areas of strength and areas needing improvement. This can help in ongoing agent coaching, training, and development.
- Real-time Monitoring: AI can monitor calls in real-time and provide immediate alerts or suggestions to supervisors if it detects issues during a live call. This enables proactive intervention when necessary.
- Agent Self-Coaching: With the help of AI, QA software uses post-call surveys and QA data to identify coaching opportunities and generate AI CX improvement coaching tips, which are displayed on the dashboard scorecard and coaching tool so that agents can self-coach to improve QA and Csat scores.
- Cost Savings: Automating QA processes with AI can save time and resources compared to manual evaluations, allowing call centers to scale their QA operations more efficiently and effectively.
However, it's important to note that while AI can significantly enhance call center QA and compliance processes, it may not completely replace the need for human involvement. Human QA experts are still essential for nuanced evaluations, making judgment calls, and providing context that AI may not fully grasp. A combined approach, where AI assists human QA teams, often yields the best results in terms of both efficiency and accuracy.
Ways That AI Can Be Applied to Automate Post-call Surveys to Integrate the Voice of the Customer Into Call Center QA
Post-call surveys can complement call center QA, but post-call surveys are not typically a substitute for QA, nor should QA be a substitute for surveys. Most call center professionals are of the view that the combination of post-call surveys and QA is the best practice to improve Csat.
Conversational IVR survey methods can be a cost-effective and effective approach for integrating the voice of the customer into call center QA processes to ensure that agents and management are customer-centric in handling calls. Incorporating post-call surveys has a proven track record for helping call center QA have a positive impact on improving Csat.
Post-call surveys and QA processes serve different purposes, with post-call surveys primarily focusing on agent Csat performance, while QA processes focus on agent call handling and compliance performance. Using both methods together can provide a more comprehensive understanding of agent and call center performance and identify opportunities to improve.
At a minimum, post-call surveys should be used to validate that QA sentiment and performance metrics positively impact Csat. However, the best practice is to conduct post-call surveys using a quota of 5 to 25 surveys per agent per month. The post-call surveys should be part of the call center QA process to determine the agent's overall QA performance and for coaching purposes. Here are some ways in which AI can be applied to automate post-call surveys and used with call center QA:
- Speech Recognition: Use automatic speech recognition to transcribe the call-recording survey conversation into text. Once the call recording is converted to text, it can be used to identify reasons for FCR, non-FCR, call resolution, unresolved calls, customer satisfaction, or dissatisfaction.
- Sentiment Analysis: Apply sentiment analysis algorithms to evaluate the emotional tone of the conversation. Automatically categorize responses as positive, neutral, or negative to gauge CX. Customer sentiment can help determine CX and assist in flagging calls for service recovery and opportunities.
- Natural Language Processing: Utilize NLP techniques to extract insights from customer comments and feedback. Analyze the text data using various NLP libraries and tools (e.g., Microsoft, Google, ChatGPT, or custom-built models). Create visualizations, such as word clouds, bar charts, quad maps, or heat maps, to provide a clear overview of the most common topics or sentiments in the survey responses.
- Conversational IVR/IVA Survey: Conversational IVR/IVA survey method is a human-like voice interaction approach for conducting surveys. It uses language models that can capture post-call customer feedback to provide valuable insights into the call center's Csat, FCR, and NPS performance and identify improvement opportunities. Conversational IVR/IVA surveys can replace human agents for conducting post-call surveys while maintaining high-quality surveys at a substantially lower cost.
- Chat Bot Conversational AI Survey: Conversational chat survey method using language models can capture post-chat customer feedback to provide valuable insights into the chat touchpoint Csat, FCR, and NPS performance and identify improvement opportunities. Make sure your chat bot can handle both text and voice interactions, as some users may prefer to provide feedback verbally.
- Real-Time Feedback: Provide customers with the option to give feedback after the call or chat through voice commands or keypad inputs. AI can process real-time feedback and escalate urgent issues based on unresolved calls or dissatisfaction criteria.
- Data Integration: Integrate post-call survey data with QA and compliance data sources in a single QA platform such as mySQM™ Customer Service QA software to gain a comprehensive understanding of the customer's journey. Use AI to integrate survey metrics with QA and compliance metrics at the agent and call center level.
- Automated Reporting: Generate automated QA scorecard reports and dashboards summarizing survey results, trends, and benchmarks. Use AI to tag non-FCR and satisfaction call reasons to identify areas of improvement and recommend actionable insights at the agent and call center level.
- Agent Self-Coaching: With the help of AI, QA software uses post-call surveys and QA data to identify coaching opportunities, which are displayed on the dashboard scorecard and coaching tool so that agents can self-coach to improve QA and Csat scores.
- Continuous Learning: Implement machine learning models to learn from customer feedback over time. Use this learning to improve call center processes, agent training, and customer service.
By leveraging AI, call centers can automate post-call surveys, gain deeper insights into customer experiences, and make data-driven decisions to enhance customer service and overall business operations.
Transforming Quality Assurance For Improving Call Center Csat
We started this blog with the well-accepted belief that call center QA is broken and has little or no impact on improving Csat, so it is only fitting to end this blog on a new Customer Service QA model that has a proven track record for improving Csat in a cost-effective manner. It is a perfect model for a call center that provides customer service support. It starts with eliminating your manual and subjective antiquated QA management system and implementing a new Customer Service QA model with AI automation features such as:
- Score 100% of customer interactions so you can take action to improve your customer satisfaction and eliminate compliance risk.
- Combine AI sentiment analysis with customer post-call survey feedback to determine the right QA metrics to use on the agent scorecard to help maintain or improve Csat. Ensure QA metrics are strongly correlated to creating high Csat and post-call survey data is strongly weighted in total QA score.
- Automated integration of post-call survey, QA, and compliance data into a single QA software platform to get holistic and transparent scores for your most important metrics, such as Csat, call resolution, AHT, sentiment, and compliance data.
- Generate automated QA scorecards and dashboards summarizing post-call survey, QA, and compliance data results, trends, and benchmarks.
- Automatically tag non-FCR and satisfaction call reasons to identify areas of improvement and recommend actionable insights at the agent and call center level.
- Implement machine learning models that can learn from customer feedback over time. Use this learning to improve call center processes, agent training, and customer service.
- Continuously calibrate your QA program to ensure alignment with business needs and customer experience.
- Utilize AI to generate agent self-coaching suggestions to improve Csat improvement based on post-call surveys and QA data.
Below is an example of an agent mySQM™ QA scorecard for a customer support call center. The scorecard is a holistic approach to evaluating calls because it includes QA, compliance, and post-call survey metrics. The data evaluation methods utilize analytical tools (e.g., CRM, ACD), artificial intelligence, and post-call surveying. The mySQM™ QA scorecard weighting is skewed toward call resolution metrics due to the fact that resolving their inquiry or problem is the most important MoT for them.
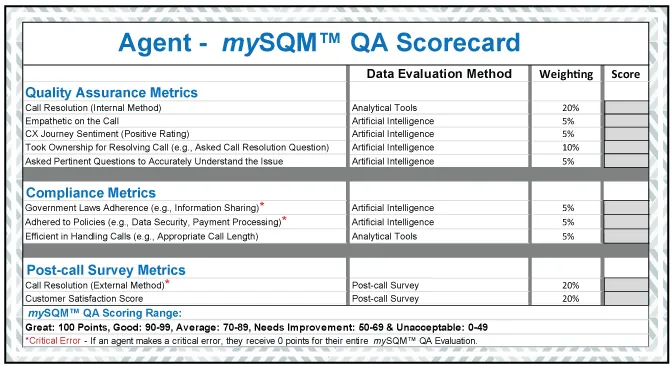