Sentiment analysis is pivotal in elevating customer experience by providing real-time insights into customer emotions. It helps by quickly figuring out how customers feel during phone calls so the team can fix any issues immediately and keep things positive. This on-the-spot feedback instantly lets agents change their approach, making the conversation more personal and understanding.
Sentiment analysis also helps monitor overall customer satisfaction so the whole team can make informed decisions to improve continuously. It's not just about solving problems faster; it also provides actionable insights for training agents to communicate better, creating an environment where customers feel understood and happy with their customer service experience.
For example, a customer contacts a tech support hotline about internet issues. With sentiment analysis, the system can detect the customer's frustration in real-time and alert the agent. This prepares the agent ahead of time and leads to a smoother customer interaction, allowing them to respond empathetically and swiftly address the technical problem on the call.
The difference between post-call surveys and sentiment analysis is that post-call surveys are solicited feedback and sentiment analysis is unsolicited feedback. This means that the feedback from post-call surveys is directly expressed and more focused because it stems from answering specific questions. The feedback from sentiment analysis needs to be determined through tone of voice and word choices.
The advantage of sentiment analysis over post-call surveys is that sentiment analysis can be performed on 100% of calls rather than a select few. This gives call centers more accurate data on agent performance and customer satisfaction.
What Is Sentiment Analysis?
Sentiment analysis is a natural language processing (NLP) technique that evaluates and interprets the emotions, attitudes, and opinions expressed in text or speech. Using algorithms and machine learning, sentiment analysis analyzes the context and language used to determine whether the expressed sentiment is positive, negative, or neutral.
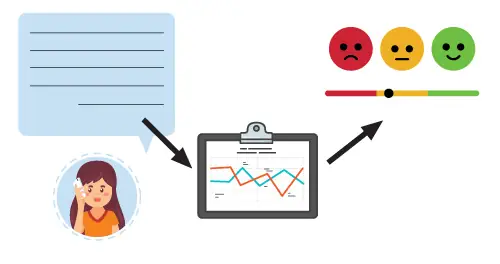
Sentiment analysis can be used in customer service to gain insights into public opinion, customer feedback, and emotional tone. Organizations can make informed decisions, enhance customer experiences, and respond effectively to the prevailing attitudes and emotions expressed in text or voice data by automating the understanding of sentiments.
Sentiment analysis can detect a customer's emotion before they are even connected to an agent. For example, a customer calls a support line and, when asked by the conversational IVR survey what the reason for their call is, the customer says, "I've been waiting for my order for weeks, and it's causing a lot of inconvenience." Sentiment analysis would pick up on text and voice data, such as negative words like "inconvenience" and a frustrated tone.
The sentiment analysis software alerts the agent of the customer's sentiment before they are connected with the agent or even while they are connected. This allows the agent to prepare themselves for the call, whether that means taking a deep breath and focusing on being patient or recalling empathy statements they could use.
How Does Sentiment Analysis Work?
Sentiment analysis works by analyzing the text of customer interactions for specific words, tones, and expressions that indicate sentiment. The process involves three main steps:
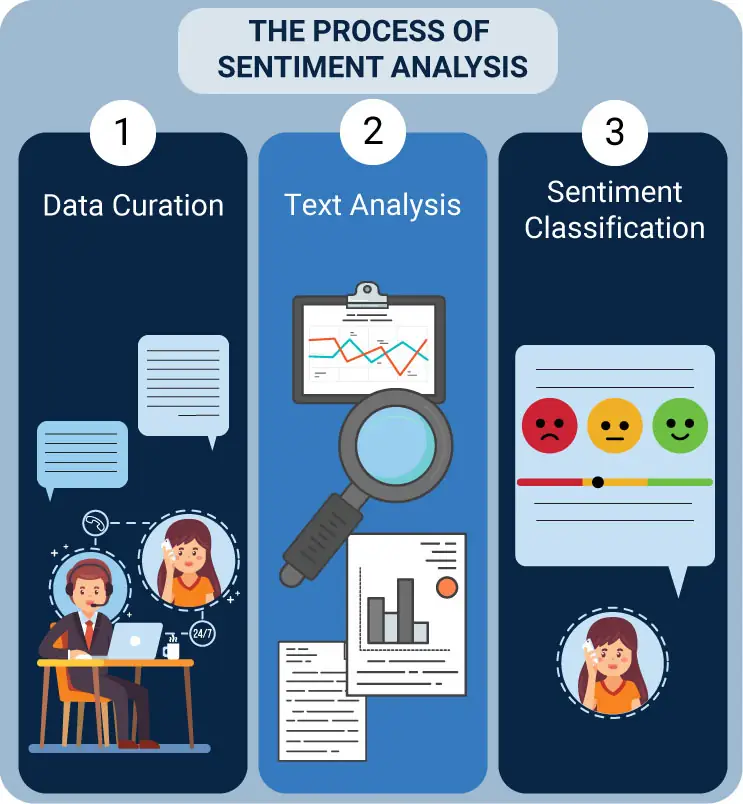
- Data curation: Transcriptions of calls or chats are pulled together.
- Text Analysis: The data is examined for words, phrases, or tone of voice cues that signify sentiment.
- Sentiment classification: The extracted sentiments are categorized as positive, negative, or neutral.
Natural Language Processing (NLP) plays a crucial role in sentiment analysis. NLP is a branch of artificial intelligence that oversees the interaction between computers and human language. It enables the system to understand, interpret, and generate text in a human-like way, thus making it possible to score and classify the sentiments in customer interactions. This process allows call centers to understand their customers' sentiments, leading to improved customer experience.
An example of this process is:
- A customer calls and says, "This is the third time that I am having issues with my computer this week. It's ridiculous!"
- Sentiment analysis uses NLP to process this speech and understand its meaning.
- Sentiment analysis then classifies this speech as negative due to the frustrated tone of voice and negative words such as "issues" and "ridiculous."
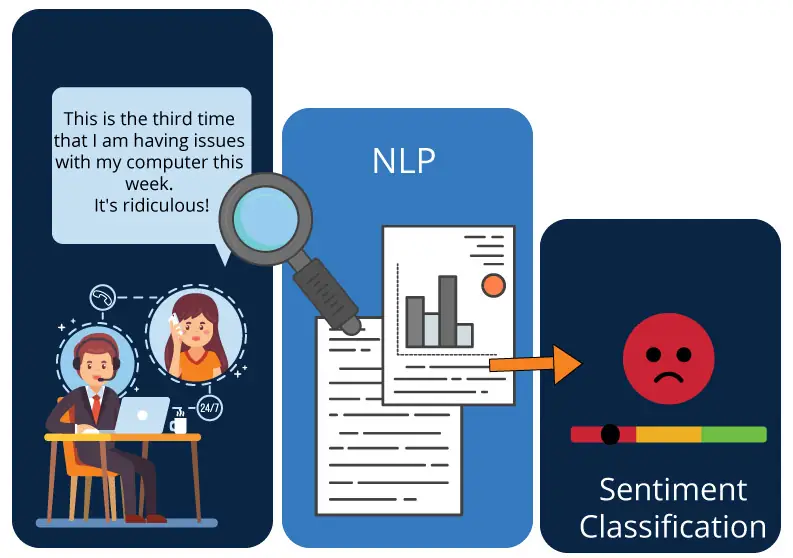
For example, after sentiment analysis detects negative words through speech data analytics, an agent could be alerted that the customer on the line is frustrated, and they can better prepare themselves to handle a frustrated customer. This lets the agent enter the call with an empathetic and helpful attitude. Sentiment analysis can also help with triaging. When someone sounds frustrated or impatient, their call could be prioritized as more urgent.
Benefits of Sentiment Analysis in Call Centers
By deciphering customer sentiment, call centers can achieve a deeper understanding and connection with their customers and their needs.
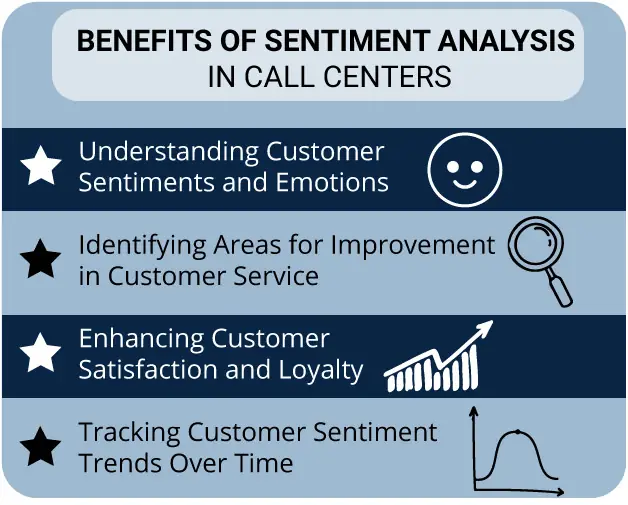
Understanding Customer Sentiments and Emotions
Sentiment analysis provides a window into customers' emotions and sentiments, allowing call center agents to understand their needs and preferences better. This understanding empowers them to connect with customers on a deeper level, improving customer experience.
For example, a customer calls a travel agency to inquire about a canceled flight. Through sentiment analysis, the call center quickly identifies the customer's frustration in their tone of voice. This understanding allows the agent to approach the conversation with empathy, addressing the emotional aspect alongside providing information about alternative options, creating a more supportive customer experience.
Identifying Areas for Improvement in Customer Service
Sentiment analysis goes beyond mere surface-level feedback. Sifting through vast customer feedback data allows you to identify recurrent themes and patterns. These actionable insights assist in pinpointing specific areas where customer service can be improved, ensuring your customers receive nothing short of exceptional assistance.
For example, sentiment analysis reveals a consistent trend of negative sentiments in customer feedback related to a specific product inquiry process. Call center managers can use this insight to investigate and revamp the training program for agents, ensuring they are better equipped to handle inquiries about that particular product, ultimately improving the overall customer service experience.
Enhancing Customer Satisfaction and Loyalty
Happier customers translate to loyal customers. Call centers can tailor their interactions to customer sentiments by applying sentiment analysis, enhancing customer satisfaction. High levels of satisfaction invariably foster brand loyalty, converting one-time customers into lifelong advocates for your business.
For example, a customer expresses delight during a call with an agent about how efficiently their issue was resolved. Sentiment analysis recognizes the positive sentiment and helps the call center team identify the key factors contributing to this customer's satisfaction. This feedback can then be used to reinforce those positive aspects in future interactions, fostering customer loyalty.
Tracking Customer Sentiment Trends Over Time
By analyzing customer sentiments over time, sentiment analysis tools enable you to track evolving customer expectations and trends. This data empowers call centers to stay ahead of the curve, tweaking customer service to align with changing customer sentiments and solidifying the business's reputation as a customer-centric organization.
For example, over several months, sentiment analysis of customer interactions reveals a growing trend of dissatisfaction related to delayed response times. This long-term tracking allows call center management to implement changes in workflow, staffing, or technology to address the issue, ensuring that customer sentiment improves over time and the call center becomes more responsive to customer needs.
Implementing Sentiment Analysis in Call Centers
Implementing sentiment analysis in call centers involves a series of critical steps, each playing a key role in enhancing the customer experience.
1. Collecting and Storing Customer Feedback Data
The first step entails collecting customer feedback through various channels such as surveys, social media comments, and call center interactions, then securely storing this vast array of data. The data could point toward the following:
- General satisfaction or dissatisfaction with the service provided.
- Specific issues and complaints they may have.
- Suggestions for service improvement
2. Choosing the Right Sentiment Analysis Tool or Software
Choosing a sophisticated sentiment analysis tool or software is essential. A suitable tool must be able to interpret complex feedback data and extrapolate customer sentiments accurately. These tools often leverage advanced technologies like AI and machine learning to identify and classify customer sentiments into positive, neutral, or negative categories.
3. Training and Integrating Sentiment Analysis into Call Center Operations
Training agents on using the chosen tool effectively and integrating it into the customer relationship management (CRM) system is vital. This system should present sentiment insights in a user-friendly interface accessible to agents in real-time. Customize the sentiment analysis algorithms to recognize industry-specific language and brand nuances, ensuring their relevance to the business context.
Integrating sentiment analysis into call center operations involves setting up real-time alerts within the system to notify agents when conversations take a negative turn, prompting immediate adjustments in their approach. Establishing a continuous monitoring and feedback loop to fine-tune the sentiment analysis system based on ongoing agent input and evolving customer interactions is also important.
Overcoming Challenges and Ensuring Accuracy in Sentiment Analysis
Sentiment analysis is a great tool to improve customer experience, but that's not to say that it doesn't come with its own challenges. For example, sentiment analysis often falls short when it comes to sarcasm and cultural differences in communication. These may bias or trick sentiment detection.
Here are some common challenges with sentiment analysis:
- Sarcasm:
Consider the statement, "Nice going!" Depending on the context, this could be genuine praise or sarcastic criticism. If someone makes a mistake and receives the response "Nice going!" with a sarcastic tone, a sentiment analysis model needs to understand the irony involved.
Advanced models can analyze the surrounding text, including punctuation, context, and historical interactions, to accurately identify the intended sentiment.
- Cultural Differences:
Cultural nuances significantly impact sentiment expression. In some cultures, expressing dissatisfaction directly might be considered impolite, leading to the use of subtle language. For example, saying "It's okay" might indicate dissatisfaction in some cultures where people tend to understate their feelings.
A sentiment analysis model trained primarily on data from a different cultural context might misinterpret such expressions. To overcome this, models should be exposed to diverse datasets encompassing various cultural communication styles, allowing them to recognize and adapt to these differences.
- Regional Expressions:
Expressions and slang terms specific to certain regions can also pose challenges. For instance, the phrase "That's wicked!" might be commonly used in some regions to express enthusiasm or approval, whereas in others, it might be associated with negativity.
Models need to be attuned to regional variations to decipher sentiments in context accurately.
- Humor and Irony:
Sentiment analysis encounters challenges with humorous content and irony. A statement like "I love spending my weekends doing chores" might be an attempt at sarcasm or humor, and a model needs to understand the underlying sentiment.
Analyzing language cues, including exaggerated language or incongruent statements, can help identify such instances.
- Hidden Opinions:
Another potential issue with sentiment analysis is that customers may not always express their true opinions to the agent. Many customers want to be polite and agreeable while speaking to the agent regardless of whether they are satisfied with the customer service. This means that sentiment analysis will not pick up on negative or positive sentiments based on their tone of voice or word choice.
In situations like these, post-call surveys are more beneficial because customers will often express their honest opinions when they are prompted to by someone else. For example, a customer usually won’t have any problem expressing their dissatisfaction if another agent asks them about their experience on a previous call.