Understanding Call Center Analytics
Call center analytics refers to the process of collecting, analyzing, and interpreting data generated from call center operations. This data can be collected from various channels, including call logs, customer interactions, social media, emails, and chat transcripts. The purpose is to gain insights into your call center by analyzing trends in the data that would otherwise go unnoticed by the naked eye.
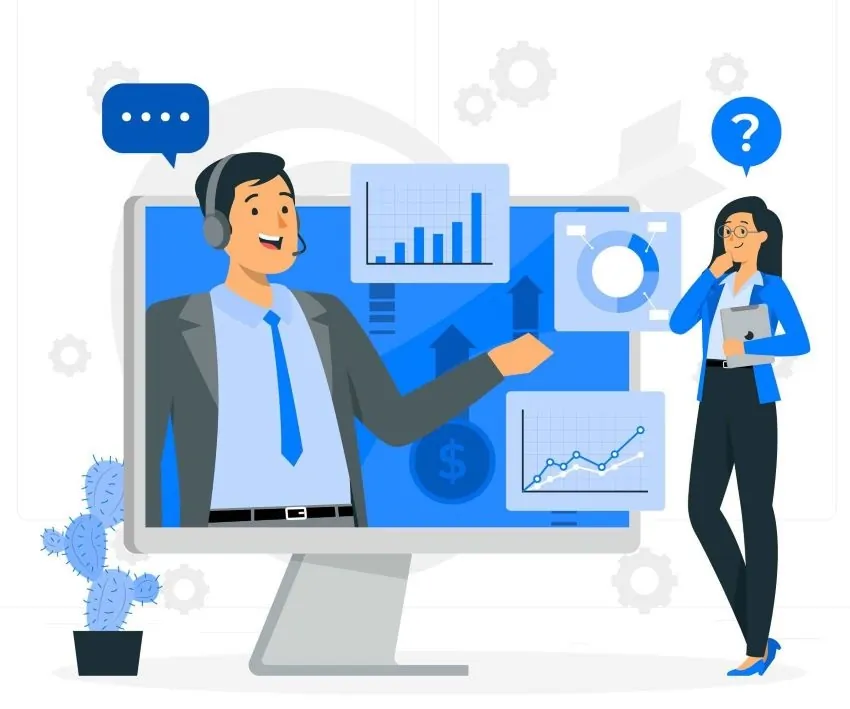
For example, consider a scenario where a financial services company operates a call center to handle customer inquiries and transaction support. Recently, the company has been experiencing a steady decline in customer retention and an increase in customer complaints about unauthorized transactions. They are perplexed by the trend and want to get to the root of the issue effectively.
In this scenario, the company could use data analytics to help discover a trend so that it can quickly take the necessary measures to fix the issues. The call center analytics solutions for this case could include the following:
- Anomaly Detection: Using statistical techniques and machine learning algorithms, the company can detect anomalies and unusual patterns in the data. The company could find correlated call data with other data sources, such as transaction logs which might provide a more comprehensive understanding of the issue.
- Predictive Analytics: By leveraging historical data and employing predictive analytics, the company can forecast potential future fraudulent activities. This allows them to take proactive measures, strengthen security protocols, and implement fraud detection mechanisms to prevent future incidents.
- Interaction Analytics: This focuses on the interactions between the agents and the customers. It can be used to evaluate agent performance and identify any unusual trends in call handling or discrepancies in issue resolution rates. This could help pinpoint whether specific agents may be involved in fraudulent activities.
There are many other scenarios where call center data analytics are highly useful in providing actionable insights which improve operational efficiency and customer satisfaction.
Types of Call Center Analytics
1. Predictive Analytics:
Call center predictive analytics leverages historical data to forecast future trends, call volumes, and customer behaviors. By identifying potential challenges in advance, call centers can proactively allocate resources, schedule staff, and optimize their operations.
For example, an insurance company wants to identify and focus on leads with the highest likelihood of converting into policyholders. It uses predictive modeling to assign a lead score to each potential customer. The model considers various factors, such as historical data on conversions, customer engagement levels, and the type of policies inquired about.
By using predictive analytics, this company can prioritize high-scoring leads for follow-up calls and give them more personalized attention to increase the chances of conversion. By doing this, the company enhances customer experience and increases sales opportunities.
2. Speech Analytics:
Speech analytics involves transcribing and analyzing call recordings to extract valuable insights from customer-agent interactions during phone calls. It can identify sentiment, emotions, keywords, and patterns in customer interactions, enabling supervisors to recognize agent training needs and spot customer pain points.
When analyzing large volumes of calls, speech analytics can help identify the root causes of customer complaints or issues. It can highlight recurring problems that may need attention to enhance customer satisfaction.
3. Customer Experience Analytics:
This branch of call center analytics revolves around measuring and improving customer experience. By analyzing customer feedback, survey responses, and call center interactions, businesses can identify areas of improvement and take corrective actions to boost customer satisfaction.
As an example, a tech company call center collects customer feedback after each interaction through post-call surveys or follow-up emails. The feedback includes ratings, comments, and specific details about the customer's experience during the call.
Customer experience analytics employs sentiment analysis to gauge the emotional tone of customer interactions. It can also identify recurring issues or frequently asked questions raised by customers. This information can be used to create knowledge base articles or FAQs, empowering agents to provide faster and more accurate solutions.
4. Interaction Analytics
Interaction analytics in a call center refers to the process of analyzing customer-agent interactions across various communication channels. The main goal of interaction analytics is to gain a deeper understanding of customer behavior, sentiments, and needs and evaluate agent performance.
The data is pulled from phone calls, emails, chat transcripts, and social media messages. This allows the call center to determine the most popular channels and identify areas of improvement.
Call Center Data Analytics Software
Data analytics software helps call centers process and analyze vast amounts of customer data efficiently and effectively. Without it, the data can be overwhelming, and extracting business insights that could streamline operations can be much more challenging.
One of the significant advantages of using call center analytics software is data visualization. Dashboards are a great example of this as they give a bird's eye view of the call center data and activity.
Call Center Dashboards
A call center dashboard uses data visualization tools such as gauge chart widgets (e.g., FCR, Csat, and action alerts). In addition, color coding (e.g., red, yellow, and green) allows users to quickly review the dashboard to determine their customer service QA performance and quickly identify areas they need to improve.
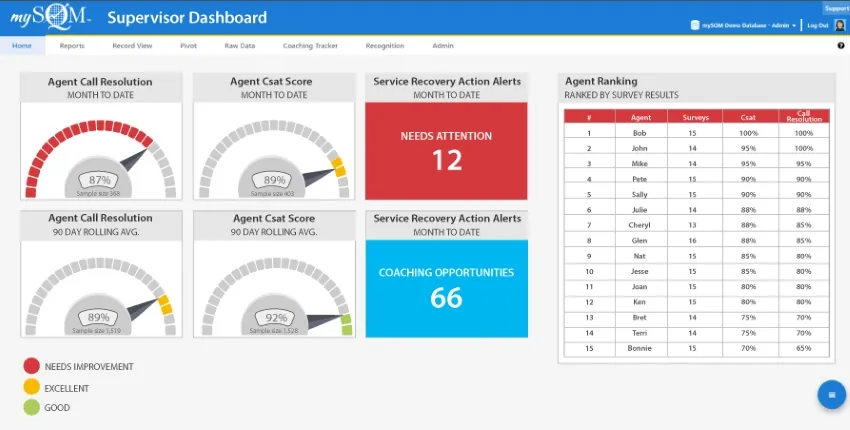
Request a mySQM™ Customer Service QA Demo Video
Learn about SQM's agent… Csat prediction model, which is based on standardized metrics, AI, and regression analysis to predict customer satisfaction derived from a QA evaluation. Our Csat prediction model provides a high statistical correlation, translating to a 95% success rate in predicting agent Csat in most cases.
Below are some of the features of an effective call center dashboard:
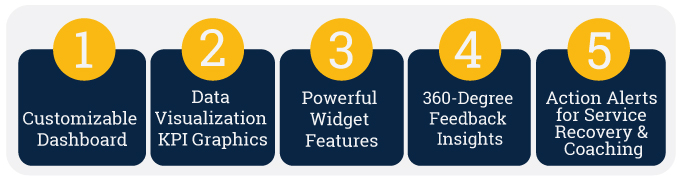
Among those that use dashboards in the call center industry, it is widely agreed that dashboards are the most crucial tool they use daily for viewing data reports and helping them improve their customer service QA KPIs and metrics.
As well as helping track and benchmark, call center dashboards are also an excellent tool for agent self-coaching. When agents can view their customer service QA performance on the dashboard, their CX performance usually increases without receiving any coaching or training. This is because dashboards provide an objective view of their performance, raising awareness and accountability.
The dashboard makes it easy for agents and supervisors to keep up to date with their CX performance. Gallup research found that employees who think their manager holds them accountable for performance are 2.5x more likely to be engaged in their job.
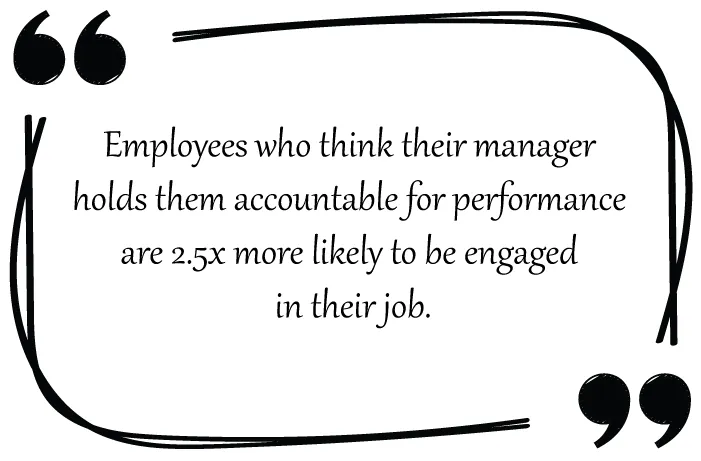
Customer Sentiment Analysis
Customer sentiment analysis uses natural language processing and machine learning techniques to analyze the emotional tone of customer interactions during calls. Based on their conversations with agents, it classifies customer sentiments as positive, negative, or neutral.
Call center software uses sentiment analysis to assess customer sentiment in real-time during calls, providing agents and supervisors with instant feedback on customer emotions. This allows agents or supervisors to respond empathetically and proactively address issues, leading to higher Csat and CX. It also allows a supervisor to step in if need be and rectify a situation before it worsens.
Why is Call Center Analytics Important?
1. Data-Driven Decision Making:
Call center analytics is crucial because it enables managers and supervisors to make informed choices based on accurate and reliable data insights. This data-driven approach allows call centers to optimize resource allocation, enhance CX, improve agent training, and streamline operations, ultimately leading to higher Csat and better overall performance.
2. Enhanced Customer Experience
Call center analytics helps identify customer preferences, pain points, and trends by analyzing customer interactions and sentiments. This enables call centers to tailor their services, address customer needs proactively, and deliver a more personalized and satisfactory customer experience.
3. Agent Performance Optimization
By allowing agents to have an objective view of their CX performance through dashboards, increases agent awareness and accountability and, by association, optimizes their CX performance as well.
4. Cost Savings and Efficiency
Call center analytics enables efficient resource planning and staffing by analyzing call data and forecasting call volumes. This prevents overstaffing during slow periods and understaffing during peak times, resulting in cost savings and optimal utilization of resources.